The stress of a pandemic is not an excuse to abandon rationality and the scientific method. In April of 2020, decades of mask research had led Fauci et. al. to tell us not to bother with masks. Yet just a few weeks later we were told to always wear masks everywhere. For anyone who was looking at research evidence, it felt like the medical establishment, press, and politicians were in a big rush to produce and promote “evidence” that masks worked.
There is a hierarchy of evidence in medical science which is well established and accepted. You know that old saying: opinions are like… ahem… noses. Everybody’s got one. That is also true for those of us in medicine. Just because an authoritative group or body recommends something doesn’t mean it’s correct. The medical establishment has a history of having universally accepted and recommended medications or treatments that were later found to be useless or even dangerous. The 1949 Nobel Prize in medicine was given to Egas Moniz - the man who invented the frontal lobotomy. The press and public health bureaucrats gushed over him at that time. “Because we say so” is not scientific justification. Politicians and medical officers of health should not default to using this statement to justify mask mandates. And for those of us in the medical profession, we should be humbled enough from our previous mistakes that we should not be cocksure and fervent about an issue as complex and evolving as masking science.
If you’re sufficiently interested you can read about the different types of medical research studies. Studies can be divided into animal vs. human. Observational vs. interventional. Blinded vs. unblinded. In vitro vs. in vivo. But in general, there is a “totem pole” or gradation of evidence, with double blind placebo controlled studies and meta-analyses on top.
Case Reports
Near the bottom of the evidence hierarchy is a “case report” – in non-medical parlance we call this an anecdote.
Let’s take a hypothetical non-COVID example. A physician tried bioflavonoids on a patient with prostate cancer, and the patient did well. The physician writes up a report, which is published in a journal. The treatment may not have actually done anything useful: maybe the patient would have gotten better anyway. But maybe this doc is on to something. A case report may spur other docs to start trying the treatment, or even spark a larger study.
Let’s take a fictional example of a COVID masking case-report. Say my friend works closely with the public as a masseuse. He and his partner both develop sniffles, which they attribute to allergies. They stay at work for a few days, and neither wears a mask. Later they test and find out their sniffles are actually due to COVID. Despite thorough contact tracing, not one of our masseuse’s contacts contract COVID. Does this prove that not wearing a mask prevents COVID from spreading? No. Of course not. This is just one story – an anecdote – and doesn’t “prove” anything, even though it’s interesting.
Now if I said the same patient and his partner DID wear masks and no contact got COVID. Did I prove that masks DO work? The answer is still no – this remains an anecdote. To be scientific, you would need compare a group of people who wore masks to similar people who didn’t wear them in similar situations, and look for differences in rates of COVID transmission to their contacts. But if you are the CDC, this type of anecdote (which would get a failing grade for a grade 7 science project) is good enough “proof” to use as a reference to say that masks definitely work. (see the infamous hairdresser study which, despite criticism, remains on the CDC website).
This study is a great example of “confirmation bias”. Although it is at best interesting, those who fervently believe masking works continue to tout its conclusions.
Surveys
Surveys are potentially more powerful than anecdotes as they have more subjects, and therefore are statistically more robust. But they have huge potential for bias. They are observational, not interventional. (See next section for why observational studies are prone to bias.) Plus, there is a huge problem of response bias. Let me explain.
If I send out 100 surveys asking if I am a good doctor, and I get 4 responses back that all say I rock, I could write a headline saying “100% of respondents think Milburn is a medical god!”. The headline is actually true. But perhaps 96% of people think I am awful, or were unable to respond because they died soon after I cared for them. Because they didn’t respond, there is no way to know what they would have said. Survey data generally sucks, and should be looked at very skeptically.
(As an aside, an organization that I am part of recently did outreach to connect with and discuss members’ concerns. They had about a 1.5% response rate. But they did not announce that the outreach was a failure, apologize, and try again. Instead they wrote a report of “what our members are telling us”. Interesting they could actually know that, given that 98.5% of members did not speak with them.)
Any survey about masking and COVID has an obvious bias. Someone who wears masks religiously, got COVID, but believes in and supports wearing masks would not want to reply. Someone who wears masks religiously and didn’t get COVID would. Someone who thinks masks are stupid will likely hang up when they hear the voice on the phone say “Hi, I’m a researcher doing a study on masks for COVID...”. “Click”.
But as with case reports like the hairdresser study, the CDC has touted survey data as “proof” for masking, including the above piece of research. Vinay Prasad does a great job of detailing the deficiencies of this study. Response rate was only about 10%. (And that is not even the worst of it if you take the time to read Dr. Prasad’s critique.)
Other observational research
“Observational studies do not involve an “intervention” but rather look at what is happening in a population and gather data from that. A survey is one type of observational research, but there are other ways of gathering data that are not dependent on response rates - for instance combing through hospital admission data, census data, etc. That said, they can still lead us astray.
Dipping into my personal “Wayback Machine Medical Memory Bank” (I’m old enough for that now) a great example of how misleading observational studies can be is the story of hormone replacement therapy (HRT) for menopausal women.
I started med school in 1993. At that time several large observational studies looked at rates of certain diseases in those who used HRT vs. those who did not, and the data was clear. Women on HRT had significantly lower risk of heart attacks, stroke, and several other medical problems. I was taught emphatically that menopause was unnatural. I was told that the human body was not designed by evolution to live that long, so living beyond menopause was an unnatural state. Rather than the menopausal fall in estrogen being a normal and natural process, it was supposedly a dangerous thing that had to be remedied with drugs. A doctor who didn’t prescribe HRT to every menopausal patient was a bad doctor, I was told.
Observational data is, however, prone to confounders, or “co-variables”. For instance, observational data shows that smoking “causes” liver failure. But it doesn’t. Alcohol causes liver failure. But alcoholics are far more likely to smoke than the general public. So people in liver failure are far more likely to be smokers. This issue of confounding is responsible for many correlations.
Eventually better research was done on HRT. It turned out that the women who were choosing to go on HRT were also the women who were choosing not to smoke. They were thinner. They exercised more. They chose a chopped kale salad at Wholefoods rather than The Baconator at Burger Thing. And when you “normalized” or adjusted the data for these factors, the apparent benefit of HRT disappeared. And a slight increase in breast cancer rate appeared, likely due to hormone stimulation. Whoopsie! Sorry about that, whole generation of women! Our bad!
So of course the medical profession learned a valuable lesson and never again jumped to conclusions...
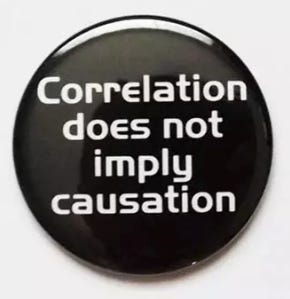
Just yanking your chain! Of course that’s not true. Spurious correlations and faulty data has remained a major problem in medicine.
And with masking, the CDC and other organizations went right back to using observational data without considering confounders. And just as observational data “proved” HRT reduced heart attacks, observational mask data “proved” that masks prevented people from getting COVID.
But here is the problem. People who are scared of COVID are more likely to voluntarily mask. They are the same people who will more likely avoid crowds, go out as little as possible, wash their hands fastidiously, and lock their children in the trunk of their car if they might have COVID. They may spend weeks at a time hiding under their bed with a tinfoil hat, praying to St. Fauci. So if people who wear masks are less likely to get COVID, that may be due to confounders, and nothing to do with actual masks. Vinay Prasad does a great job of explaining this in the video below.
RCT’s – randomized controlled trials
The gold standard of research - sitting at the pinnacle of medical science, gleaming in the sun – is the “Randomized Controlled Trial” or RCT. An ideal study has half of the group take sugar pills and half take the real drug. In a blinded study, the patient doesn’t know which they are getting. This prevents the placebo - or nocebo - effect from skewing the data. A “double-blind” trial means neither the patient nor the doctor knows if the patient is getting the real treatment or placebo. This prevents the doctor from skewing the data, subconsciously or otherwise. A double-blind RCT is the best we can do in medical science.
Obviously, a mask study can’t be blinded for the patients. People know if they are wearing a mask. But it can be randomized. Pick 1000 people. Randomly assign 500 to wear masks and 500 not to. In a few months, see who got COVID. (It can also be blinded in the data analysis – but that is a different discussion and overly technical for my purpose here).
There were 2 significant RCT’s done on masks.
Danmask
One was done in Denmark. It followed several thousand people through several months. The conclusion? Masks made very little, if any difference. The British Medical Journal (one of the braver medical research outlets, that actually was willing to argue with accepted wisdom) published a critical take that I quite agree with. If you want to get really down, dirty, and get your geek on regarding what is known as the “Danmask” study, you can check out either this discussion or this one.
Remember that issue of absolute (ARR) versus relative (RRR) risk reduction I discussed in a previous post? Recall that RRR is the favoured statistic used when someone needs to sell useless or marginally useful products. Well, the Danish study showed a possible RRR of ~13-14%. But ARR was only about 3 in 1000 during a time of significant COVID transmission, and was achieved only by wearing your mask religiously for several months. During lower-prevalence times (ie: most of the pandemic in most of the world), ARR would be even less than 3 in 1000 over several months.
2 more caveats about Danmask.
First, the small benefit seen was for surgical masks, not cloth masks — you know, the cloth masks they forced us to wear for the last 2 years. Cloth masks logically must be much less useful than surgical masks due to the much larger pore size. So their ARR value would be much less than 3/1000 over several months of use, and as the next study I mention shows, they are likely 100% useless.
Second, for those who don’t mind getting math-y, the result was not “statistically significant” – ie: there is a reasonable chance the benefit of masks was actually zero, not 13%. If you flip a coin 6 times and it comes up heads 4 times, and tails twice, it doesn’t mean the coin is biased, it might be chance. And like the 4 heads, this study result may have been due to statistical chance, not a real effect of masks.
Bangladesh Trial
The second RCT was a VERY large study done in Bangladesh involving hundreds of thousands of subjects, which assigned certain villages to several COVID-reduction initiatives including handwashing, physical distancing, and wearing masks. The result was a very small reduction in transmission. This study was touted it in the press and social media as more “proof” that masks work. But the actual results showed that cloth masks had zero effect, and even surgical masks had only a minimal effect. (This is a fantastic critique of the data, again best for people who like to geek out.)
But this is how the media covered it:
There were significant problems with the glowing conclusions like the headline above.
First, the intervention wasn’t limited to masking. As described above, it included education on social distancing, hand washing, etc. We don’t know how much of the reduction was due to masking, and how much might have been due to other measures.
Secondly, the number of cases prevented could be counted on hands and feet, out of a study group of almost 200,000 people. Again it’s that pesky issue of ARR vs. RRR. I could say that masking decreased COVID transmission by 12%. That sounds good. Or I could tell you that months of continuous masking reduced your odds of getting COVID from 9 in 1000 to 8 in 1000. That doesn’t sound nearly as compelling. Also consider that people had to agree to be part of the study in the first place. So study participants may have been more motivated and willing to properly and fully comply with measures than the general public.
Then there is the issue of applicability. Is the Bangladesh result applicable to you? Cape Breton (where I live) has a population density of 12.7 humans per square Km, Denmark’s population density is 10 times higher and Bangladesh 100 times higher. This is important, because logically the lower the population density, the less effect masks would have. Even if one finds the Bangladesh result compelling and thinks that reducing transmission from 9/1000 to 8/1000 is worth a mask mandate there, can we really apply this universally? Most of us do not live in social circumstances and population densities similar to a Bangladeshi village.
(Vinay Prasad discusses the Bangladesh study here. I agree with his criticism, but would have gone further and stressed the very small absolute (versus relative) risk reduction issue. As well, he doesn’t mention that masks were not the sole intervention, so other factors may have caused the small difference.)
To Summarize
So just to review, because this was a long post!
Anecdotal studies were used to “prove” masks work. They prove nothing, even if they suggest the need for more research.
Survey data is extremely prone to response bias. The results of a survey may not be trustworthy enough to judge whether a restaurant is good, let alone to provide the basis for a very intrusive, destructive, and divisive universal public policy.
Observational data was touted to “prove” masks work. This type of data can be extremely unreliable and has led to some very significant medical mistakes in the past. Unless carefully controlled for confounding factors, observational trials can and do lead us astray.
RCT’s are the gold standard of medical research. But mask studies can’t be blinded, which can cause confounding. The 2 RCT’s available show minimal effect on absolute risk reduction. In the Danmask study the effect seen may be due to statistical chance, and in the Bangladeshi study the effect could be due to other co-interventions.
Public policy is not created by “following science”
Would you slavishly wear a mask for months (actually, years!) on end if you knew it made minimal, or possibly no, difference? That it would reduce your chances of catching COVID only by 1 in 1000 over months? And even if you would be OK with that, does that mean that everyone else should be forced to wear one? As I’ve discussed before, science can’t guide us, it can only inform our decisions. Forcing people to mask against their will is necessarily a political decision because it requires consideration of our values and priorities, and weighing them in the final decision.
Mask efficacy versus mask mandates
Whether mask mandates work is a subtly different question to whether masks work. A study group might have less COVID if they wear masks. But does this remain true when this is applied to the entire population?
Do mask mandates work? As I’ve discussed in this post, study data is imperfect due to biases and unavoidable research limitations. At best it can suggest that masks (and therefore mask mandates) may help, but it can’t prove they do. The best evidence we can consider for the effectiveness of mask MANDATES is to see if they work in the real world. And for that we need to step back from the individual studies, pan out and look at The Big Picture. How have areas with mask mandates fared against those without mandates? Have we seen definite decreases in COVID after mask mandates are implemented? The answer is interesting and instructive. And before our public health overlords decide that the next wave of COVID justifies a new mask mandate, we need to make sure this Big Picture data does not get swept under the carpet.
I would suggest to justify the massive infringement of our rights that a mask mandate represents, there should have been clear data to do so. It should have proved decisively that an unmasked person is a clear and present danger to others, like a drunk driver or someone waving a loaded gun around in a crowd. If this were true there would be large reductions in COVID rates in areas with universal masking versus areas without. There should have been clear changes in COVID transmission rates in an area when a mask mandate was started.
In this post I’ve endeavoured to point out that study data supporting masking was very weak. Next post will show that the proof was not evident in the pudding either.
Coming up Next: We have The Charts
For doctors, you both understand science quite well! That's not very common in your profession. Excellent write up and critique of why ARR and population/region being studied matters!
great article doc...as someone who early on in the scamdemic looked at the mask studies that showed insignificant to no benefit to masking(except to the mask producers/sellers), I feel this issue has been beat to death but just won't die...anyone can claim anything today without evidence and if there is enough money to support the issue/cause then that's what is pushed